Revolutionizing Food Security and Disaster Preparedness in Bangladesh with Satellite Technology
By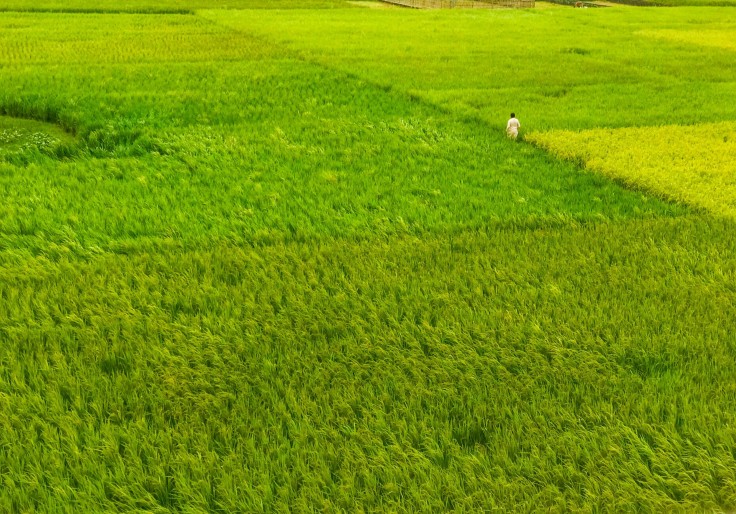
The fusion of machine learning and satellite imagery is ushering in a new era of environmental monitoring and agricultural management. This powerful combination offers unparalleled insights into the health of our crops, the risks posed by natural disasters, and the ever-changing tapestry of our planet.
Unveiling the Potential of Rice Crops in Bangladesh
A recent study by North Carolina State University (NC State) exemplifies the transformative potential of this approach. The research team focused on Bangladesh, the world's third-largest rice producer and a nation highly vulnerable to climate change. Traditional methods of monitoring crop productivity, reliant on field data collection, are laborious and time-consuming. This in turn hinders informed decision-making regarding exports, imports, and crop pricing.
To address this challenge, the researchers leveraged the power of machine learning and satellite imagery. By analyzing time series satellite imagery – a series of images captured over regular intervals – they were able to gauge vegetation health, growth conditions, crop water content, and soil conditions across vast swathes of land. This rich data stream, when combined with field data, empowered them to train a machine learning model for meticulously estimating rice crop productivity between 2002 and 2021.
The implications of this research are far-reaching. The model can pinpoint areas of high and low productivity, enabling policymakers to allocate resources strategically. For instance, surplus production in a particular region can inform decisions regarding storage capacity and transportation infrastructure development. More importantly, the availability of this information well in advance empowers policymakers to make critical choices about crop diversification, introducing climate-resilient rice varieties, and optimizing cropping patterns.
The accuracy of the model is another noteworthy aspect. It boasts a range of 90-92 percent with a 2 percent margin of error. This degree of precision paves the way for the model's adaptation to various crops and diverse landscapes. The success in Bangladesh, where rice forms the staple food for 90% of the population and agriculture contributes significantly to the GDP, underscores the model's potential for global application.
This research is a testament to the power of collaboration. Stakeholders, researchers, and policymakers from NC State, the U.S. Department of Agriculture, the International Maize and Wheat Improvement Center, and the Bangladesh Rice Research Institute joined forces to ensure the utilization of best scientific practices for informed decision-making. The publication, titled "Advancing Food Security: Rice Yield Estimation Framework using Time-Series Satellite Data & Machine Learning," in PLOS ONE, further underscores the significance of this collaborative effort.
Envisioning Floods: AI-Generated Satellite Images for Enhanced Preparedness
While NC State's research focuses on agricultural monitoring, scientists at the Massachusetts Institute of Technology (MIT) are harnessing the potential of machine learning and satellite imagery for a different purpose – predicting flood scenarios. They are developing an AI tool that generates realistic satellite images depicting potential flooding events.
This tool leverages a generative AI model, specifically a Generative Adversarial Network (GAN), in conjunction with a physics-based flood model. The GAN generates detailed bird's-eye view images of a region, portraying the potential impact of an approaching storm based on its intensity.
However, GANs are susceptible to producing "hallucinations" – unrealistic or inaccurate features within the generated images. To mitigate this challenge, the researchers incorporate a physics-based flood model. This model acts as a guiding force, ensuring the generated images align with real-world flood dynamics.
The benefits of this approach are multifaceted. Firstly, it can serve as a powerful visualization tool for policymakers. Images depicting potential flood inundation can provide a more tangible and emotionally engaging experience compared to traditional color-coded maps. This can bolster public preparedness and encourage timely evacuations in flood-prone areas.
The research team demonstrated the efficacy of their model by simulating a scenario in Houston, generating satellite images of flooding caused by a storm similar in strength to Hurricane Harvey. The AI-generated images that lacked the physics-based model integration exhibited significant inaccuracies, showcasing extensive flooding in unrealistic locations. Conversely, the images produced using the physics-reinforced method closely resembled the actual flooding observed during Hurricane Harvey.
This technology holds immense promise for the future. It can empower policymakers to develop comprehensive flood-preparedness plans, including evacuation strategies and mitigation efforts. The ability to visualize potential flood scenarios can significantly enhance decision-making processes.
The current iteration of this technology is in the proof-of-concept stage. Further research is required to refine the model's ability to predict flood outcomes across diverse regions and storm types. As Dava Newman, professor of AeroAstro and director of the MIT Media Lab, aptly states, "We can't wait to get our generative AI tools into the hands of decision-makers at the local community level, which could make a significant difference and perhaps save lives."