Evaluating the Impact: How QA Will Assess the Power of New Generative AI-Based Testing Tools
Created by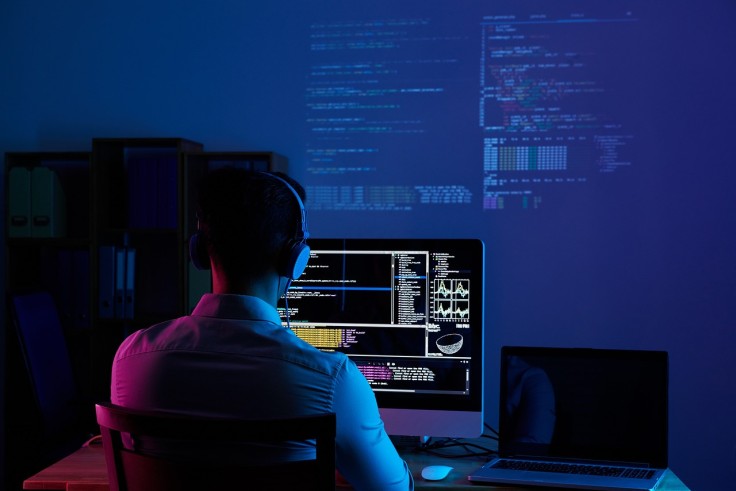
The advent of generative AI-based testing tools is poised to revolutionize the landscape of Quality Assurance (QA) in software development. As these intelligent automated QA testing tools harness the capabilities of artificial intelligence to create, execute, and adapt test cases autonomously, QA teams face the challenge and opportunity of evaluating their impact comprehensively.
Here are the ways QA professionals will assess the power and effectiveness of these new generative AI-powered testing tools:
1. Test Case Coverage and Diversity:
Traditional testing methods often struggle with achieving comprehensive test case coverage, especially in complex applications. QA teams will evaluate generative AI-based testing tools according to their ability to generate diverse and exhaustive test cases automatically. The focus will be on ensuring that the AI tools cover various scenarios, user interactions, and potential edge cases to identify hidden defects and vulnerabilities.
2. Adaptability to Dynamic Environments:
One of the key challenges in modern software development is the dynamic nature of applications and their environments. QA professionals will assess the adaptability of generative AI-based testing tools to changes in application features, user interfaces, and underlying technologies. The tools must demonstrate the capability to adjust test cases as the software evolves autonomously, ensuring continued relevance in dynamic development ecosystems.
3. Efficiency in Bug Detection and Reporting:
Generative AI-powered testing tools are expected to enhance the efficiency of bug detection and reporting. QA teams will closely evaluate how well these tools identify and prioritize defects, reducing the time and effort required for manual debugging. The effectiveness of the tools in providing actionable insights and comprehensive bug reports will be a critical metric in determining their impact on the overall testing process.
4. Reduction in Testing Time and Resource Utilization:
Speeding up the testing process without compromising quality is a significant goal for QA teams. Generative AI-based testing tools are anticipated to contribute to this objective by automating repetitive tasks and streamlining test case execution. QA professionals will assess the extent to which these tools reduce testing time and optimize resource utilization, allowing teams to focus on more complex testing challenges.
5. Alignment with Industry Compliance Standards:
Industries with stringent compliance standards, such as finance and healthcare, require adherence to specific testing protocols. QA teams will evaluate how generative AI helps these testing tools align with industry compliance standards and regulatory requirements. Ensuring that these tools provide accurate and reliable results in compliance-focused testing scenarios is essential for their adoption in regulated industries.
6. Integration with Existing Testing Frameworks:
To facilitate seamless adoption, these modern testing tools must integrate effectively with existing testing frameworks, tools, and methodologies. QA professionals will evaluate how well these tools fit into their current workflows, ensuring that the integration process is smooth and does not disrupt established testing practices.
7. Human Oversight and Collaboration:
While the autonomy of generative AI-powered testing tools is a strength, QA teams will assess the level of human oversight and collaboration that these tools allow. Ensuring that human testers can intervene, validate results, and contribute domain-specific knowledge is crucial for maintaining a balance between automation and human expertise.
Conclusion:
As generative AI becomes an integral part of the QA landscape, its impact will be evaluated across various dimensions. From test case coverage and adaptability to efficiency in bug detection and compliance with industry standards, QA professionals will rigorously assess the capabilities of these tools. The successful integration of these next-generation tools into QA processes will accelerate testing cycles and contribute to the overall quality and reliability of software applications.
The journey of evaluating the impact of these tools marks a transformative phase in QA, where the synergy between artificial intelligence and human expertise promises to redefine the future of software testing.